فهرست مطالب
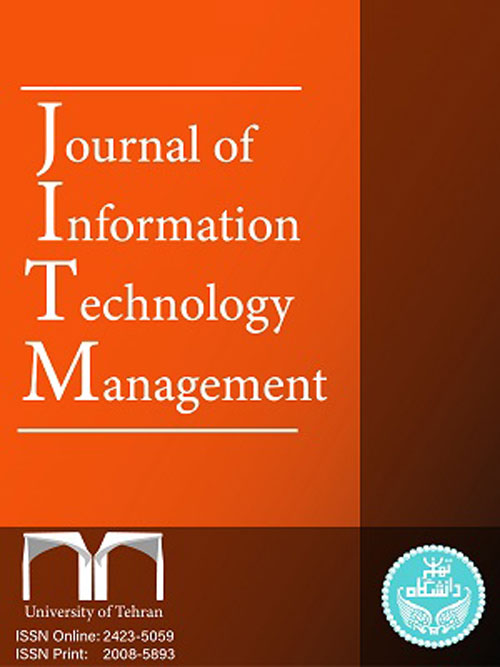
Journal of Information Technology Management
Volume:14 Issue: 4, Autumn 2022
- تاریخ انتشار: 1401/05/20
- تعداد عناوین: 8
-
-
Page 1
Internet of Things (IoT) makes our reality as conceivable as associated together. These days we nearly have a web framework in almost every place and we can utilize it at any point. Installed embedded gadgets would be exposed to web impact. Regular cases for installed embedded gadgets are MP3 players, MRI, traffic signals, microwaves, clothes washers and dishwashers, GPS, and even heart checking inserts or biochips and so on. IoT tries to set up cutting-edge availability (with the guide of the web) among these referenced gadgets or frameworks or administrations to gradually make automation in all regions. It is possible to picture that all things are associated with each other, and all data would be linked to one another over a standard and distinctive convention space and applications. The Industrial Internet of Things (IIoT) or the fourth Industrial insurgency or Industry 4.0 are generally names given to the utilization of IoT innovation in a business setting. The idea is equivalent to implementing IoT gadgets in the home, yet for this situation, the point is to utilize a combination of sensors, remote systems, enormous information, AI, and investigation to quantify and streamline modern procedures. Instead of simply singular organizations, if presented over a whole supply chain, the effect could be significantly more noteworthy with quick conveyance of materials and the administration of creation from start to finish. Expanding workforce efficiency or cost reserve funds are two expected points, yet the IIoT can likewise make new income streams for organizations instead of simply selling an independent item – for instance, like a motor – makers can likewise sell predictive service of the motor.
-
Pages 2-18In recent scenario, women are suffering from breast cancer disease across the world. Mammography is one of the important methods to detect breast cancer early; that to reduce the cost and workload of radiologists. Medical image processing is a tremendous technique used to determine the disease in advance to reduce the risk factor. To predict the disease from 2-D mammography images for diagnosing and detecting based on advanced soft computing paradigm. Still, to get more accuracy in all coordinate axes, 3-D mammography imaging is used to capture depth information from all different angles. After the reconstruction of this process, a better quality of 3D mammography is obtained. It is useful for the experts to identify the disease in well advance. To improve the accuracy of disease findings, deep convolution neural networks (CNN) can be applied for automatic feature learning, and classifier building. This work also presents a comparison of the other state of art methods used in the last decades.Keywords: Breast Cancer, Mammography, Radiologists, CAD, Deep learning, Convolutional Neural Network, Medical imaging
-
Pages 19-39Android malware is one of the most dangerous threats on the Internet. It has been on the rise for several years. As a result, it has impacted many applications such as healthcare, banking, transportation, government, e-commerce, etc. One of the most growing attacks is on Android systems due to its use in many devices worldwide. De-spite significant efforts in detecting and classifying Android malware, there is still a long way to improve the detection process and the classification performance. There is a necessity to provide a basic understanding of the behavior displayed by the most common Android malware categories and families. Hence, understand the distinct ob-jective of malware after identifying their family and category. This paper proposes an effective systematic and functional parallel machine-learning model for the dynamic detection of Android malware categories and families. Standard machine learning classifiers are implemented to analyze a massive malware dataset with 14 major mal-ware categories and 180 prominent malware families of the CCCS-CIC-AndMal2020 on dynamic layers to detect Android malware categories and families. The paper ex-periments with many machine learning algorithms and compares the proposed model with the most recent related work. The results indicate more than 96 % accuracy for Android Malware Category detection and more than 99% for Android Malware family detection overperforming the current related methods. The proposed model offers a highly accurate method for dynamic analysis of Android malware that cuts down the time required to analyze smartphone malware.Keywords: Android Malware, Malware Analysis, Malware Category Classification, Malware Family Classification, Malware Dynamic Analysis
-
Pages 40-56The aim of this study is to evaluate the performance of the pre-trained models and compare them with the probability percentage of prediction in terms of execution time. This study uses the COCO dataset to evaluate both pre-trained image recognition and object detection, models. The results revealed that Tiny-YoloV3 is considered the best method for real-time applications as it takes less time. Whereas ResNet 50 is required for those applications which require a high probability percentage of prediction, such as medical image classification. In general, the rate of probability varies from 75% to 90% for the large objects in ResNet 50. Whereas in Tiny-YoloV3, the rate varies from 35% to 80% for large objects, besides it extracts more objects, so the rise of execution time is sensible. Whereas small size and high percentage probability makes SqueezeNet suitable for portable applications, while reusing features makes DenseNet suitable for applications for object identification.Keywords: Deep learning, Image recognition, Object Detection, Pre-trained Models
-
Pages 57-68This paper presents the design, simulation and realization of a patch antenna for IOT applications. The patch antenna consists of a radiating element printed on one face of a dielectric substrate, when the ground plane is placed on the other face. In this work, two techniques are used to design a miniaturized patch antenna: the set-up of slots on the radiating element and the use of defective ground plane. Also, the slot’s radius and Length of inset point effects on the performances of the antenna is illustrated. All the simulated results are performed with FEKO, a solver based on a Moments Method and measurement is made using Vector Network Analyzer Anritsu MS2026C. The propose antenna resonates in three frequency bands 3.91, 4.86 and 5.16GHz for different characteristics such as radiation pattern, gain, return loss, which makes it suitable for many wireless communication applications such as IoT applications.Keywords: IOT, Patch Antenna, Slots, UWB, 5G
-
AI-WSN: Direction of Arrival Estimation Based on Bee Swarm Optimization for Wireless Sensor NetworksPages 69-86An Artificial Intelligence (AI) technique plays the most crucial factor to consider in energy utilization in a wireless sensor network (WSN). AI transforms industrial operations by optimizing the energy consumption in sensor nodes. As a result, it is crucial for improving sensor node location accuracy, particularly in unbalanced or Adhoc environments. Because of this, the purpose of this research is to improve the accuracy of the localization process in locations where sensor nodes encounter barriers or obstacles on a regular basis. The Bees Swarm Optimization (BSO) algorithm is used to segment sensor nodes in order to increase the accuracy of the Direction of Arrival (DoA) estimate between the anchor and unknown node pairs. Even in the presence of unbalanced conditions, the proposed DoA- BSO involving three separate bee colonies can identify plausible anchor nodes as well as segment nodes arranged in clusters. In order to obtain the intended result, the objective function is designed to take into consideration the hops, energy, and transmission distance of the anchor and unknown node pairs, among other factors. The studies are carried out in a large-scale WSN using sensor node pairs in order to determine the precision with which the DoA-BSO can be located. When comparing DoA-BSO to conventional approaches, the findings of the meta-heuristic algorithm show that it improves the accuracy and segmentation of nodes significantlyKeywords: Wireless Sensor Network, Direction of arrival, Bees Swarm Optimization, Energy estimation
-
Pages 87-94This study compared the performance of the partial least squares-structural equation modelling (PLS-SEM) and the robust partial least squares -structural equation modelling (RPLS-SEM) methods through Winsorisation approach The inputs and the outputs used in this model were based on the electricity generation data, derived from the Al-Zawiya Steam Power Plant, Libya. Furthermore, the researchers compared the novel RPLS-SEM approach with the traditional PLS-SEM approach and noted that the novel RPLS-SEM method was more efficient compared to PLS-SEM.Keywords: Partial Least Square-Path Modelling (PLS-SEM), Robust Partial Least Squares (RPLS-SEM), Structural Equation Modelling (SEM), Winsorization, Steam Power Plant, SmartPLS3
-
Pages 95-117The main aim of this thesis is to investigate the factors that could affect the students to adopt e-learning system in Libyan universities. This study is quantitative approach, a questionnaire was adopted from previous studies and distributed among the students to collect the data. The sample of the study consists 365 students from Libya. AMOS software was used to analysis the data. The results indicated that Performance Expectancy, Efforts Expectancy, Facilitating Conditions, Habit and Trust have significant impact on behavioural intention. Moreover, the relationship between Behavioural intention and use behaviour is also significantly positive. However, the relationship of social influence and behavioural intention was not found significant. Finally, the moderation effect was significant and supported between social influence, trust, and Habit with behavioural intention.Keywords: E-learning, UTAUT2, trust, Structural Equation Modeling, Libya